MPI SUMMER SCHOOL 2013: DECISION MAKING IN A SOCIAL WORLD. DUNCAN WATTS KEYNOTE
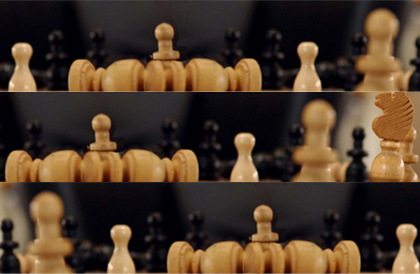
Summer Institute on Bounded Rationality
Decision Making in a Social World
This summer Gerd Gigerenzer and Ralph Hertwig will host the annual Summer Institute on Bounded Rationality, with a focus on “Decision Making in a Social World”.
Dr. Duncan Watts of Microsoft Research, author of “Six Degrees: The Science of a Connected Age”, will give the keynote address. This year marks the first time the event is jointly presented by the Center for Adaptive Behavior and Cognition as well as the Center for Adaptive Rationality at the Max Planck Institute for Human Development in Berlin.
Join renowned faculty and international participants across various disciplines for talks and workshops during the one-week Summer Institute. 35 young scholars will have the opportunity to explore how bounded rationality helps the navigation of the complex social world, as well as present their research and receive feedback from and network with distinguished researchers and fellow young scholars.
We invite talented graduate students and post-doctoral fellows to apply before April 10, 2013. Details on the Summer Institute and the application process are available at http://www.mpib-berlin.mpg.de/de/forschung/adaptives-verhalten-und-kognition/summer-institute-on-bounded-rationality.
Questions are welcomed to summerinstitute2013@mpib-berlin.mpg.de.
Feel free to pass the information on to interested (and interesting) researchers.
Filed in
Ideas
Subscribe to Decision Science News by Email (one email per week, easy unsubscribe)
HERE ARE YOUR ANSWERS
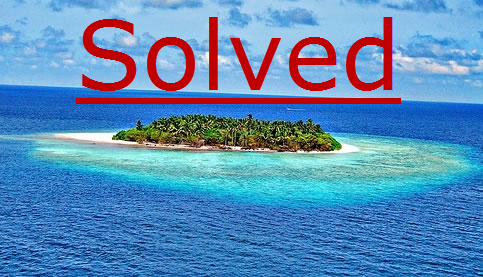
Last week we posted two fun probability problems. If you haven’t given them the old college try do so now, because this week we present you with the answer, provided by the man who supplied the problems, author and Professor of Operations Research and Probability Henk Tijms. Voila.
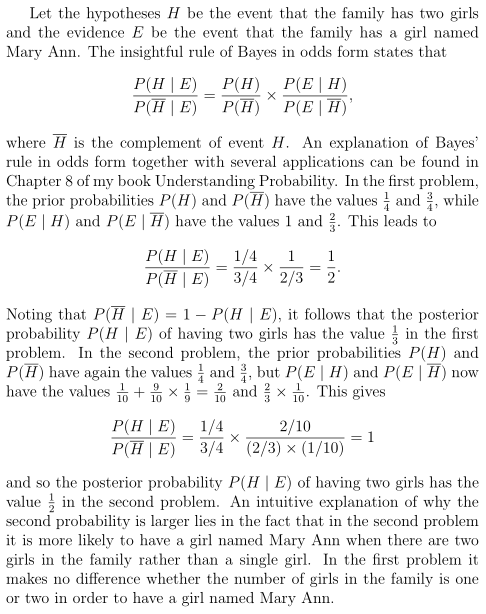
Enjoy this kind of thing? Try the Tuesday’s child is full of probability problems problem.
Filed in
Ideas
Subscribe to Decision Science News by Email (one email per week, easy unsubscribe)
COUNTERINTUITIVE PROBABILITIES REDUX
Drawn in by our post Tuesday’s child is full of probability problems, author and Professor of Operations Research and Probability Henk Tijms writes in with two new puzzles:
Problem 1:
An isolated island is ruled by a dictator. Every family on the island has two children. Each child is equally likely a boy or a girl. The dictator has decreed that each first-born girl (if any) in the family should bear the name Mary Ann (the name of the beloved mother-in-law of the dictator). Two siblings never have the same name. You are told that a randomly chosen family that is unknown to you has a girl named Mary Ann. What is the probability that this family has two girls?
Problem 2:
The dictator has passed away. His son, a womanizer, has changed the rules. For each first-born girl in the family a name must be chosen at random from 10 specific names including the name Mary Ann, while for each second-born girl in the family a name must be randomly chosen from the remaining 9 names. What is now the probability that a randomly chosen family has two girls when you are told that this family has a girl named Mary Ann? Can you intuitively explain why this probability is not the same as the previous probability?
If you need a hint, he adds this postscript:
P.S. As you know, the wording in this kind of problems is crucial. I found that the best approach to attack this kind of problems is to use Bayes’ rule in odds form. This specific form of Bayes forces you to make transparent the assumption you are (implicitly) making in solving the problem. I take the liberty to mention that in the recent third edition of my book Understanding Probability
(Cambridge University Press, 2012), I advocate the use of Bayes’ rule in odds form (and Bayesian thinking in general).
Who can solve it first?
Filed in
Ideas ,
R
Subscribe to Decision Science News by Email (one email per week, easy unsubscribe)
EVERYDAY RISKY CHOICE
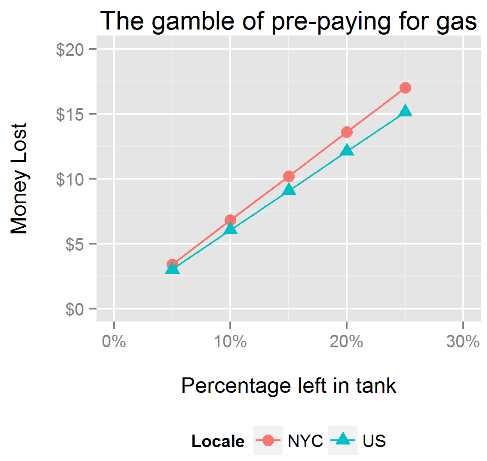
One question we get asked a lot is whether it’s worth it to pre-pay for the tank of gas when renting a car.
At first, blush it seems like something you should never do. In the best case, you pay market rate for gas, and in the worst case, you pay for a tank of gas you never consume (what if your trip gets cancelled)?
At second blush, it can be worth the risk to avoid the hassle of fueling up just before returning the car. If your time and peace of mind are worth something, then maybe you should pre-pay when you are reasonably sure you’ll return it below a certain percentage full. But what percentage?
To help with this decision, we’ve calculated the amount of money you waste when returning the car at various percentages full (above). We plugged in the New York City price of gas we face ($4) and the current national average ($3.56). For us, the hassle of refueling in the South Bronx after a weekend in the country is about $5, so we should probably pre-pay when we’re pretty sure we’ll return the car about 5-10% full.
We discussed this topic with Sid Suri and concluded that many factors go into this decision:
- Freedom from stress of having to fill up
- Stress of trying to run the tank down as low as possible without running out of gas (sure, it’s a commission of the sunk cost fallacy, but we’re humans)
- The thrill of returning the car right before it runs out of gas
- Time of day of return
- Cognitive costs of deciding how much gas to purchase during a trip so it’s nearly empty upon return
- Safety of gas station near return location
- Gas lines (we once pre-paid after Hurricane Sandy and avoided a several-hour long gas line)
- The “fee” for returning the car less than 100% full (something like $8 / gallon) vs. the expected loss by pre-paying
We think that:
- It would be much better if you could just pre-pay for a quarter tank instead of a tank. We’d even accept a small fee to do this.
- Most people who pre-pay are sticking it to their employers.
Graphs were made in R using Hadley Wickham’s ggplot2 package.
Code is here:
library(ggplot2)
library(scales)
NYC=4
NAT=3.56
pl=seq(.05,.25,.05)
gal=pl*17
nycost=gal*NYC
natcost=gal*NAT
mdf=data.frame(
Percentage_full=c(pl,pl),
Cost=c(nycost,natcost),
Locale=c(rep("NYC",length(pl)),rep("US",length(pl))))
p=ggplot(mdf,aes(Percentage_full,Cost,group=Locale,
color=Locale,shape=Locale))
p=p+geom_point(size=3)+geom_line()
p=p+scale_x_continuous("\nPercentage left in tank",limits=c(0,.3),
labels=percent_format())
p=p+scale_y_continuous("Money Lost\n",limits=c(0,20),
labels=dollar_format())
p=p+theme(legend.position="bottom")
p=p+ggtitle("The gamble of pre-paying for gas")
p
DOES IT MATTER WHERE IT IS KICKED? ANALYSIS OF OVER 10,000 ATTEMPTS
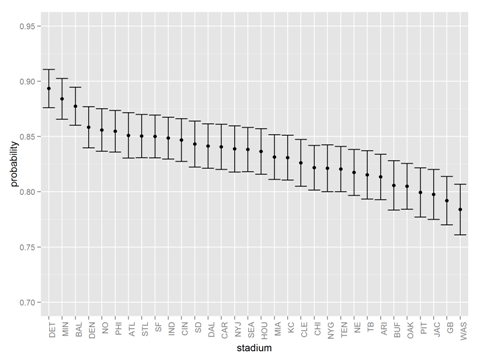
Click to enlarge
In our third of not one, not two, but three posts on kicking a football in the NFL, we take on a reader question of whether the stadium / home team matters for making a field goal. We pulled up the data on every field goal since 2002 (over 10,000) of them and plotted the probability of scoring as a function of the stadium in which the field goal was kicked. The results are above. Bars are +/- 1 standard error.
Is it a statistically significant effect? Apparently so:
Pearson's Chi-squared test
X-squared = 49.9556, df = 31, p-value = 0.01693
ADDENDUM 1
We had a request to see the broken down by home team or visiting team kicking. Here you go:
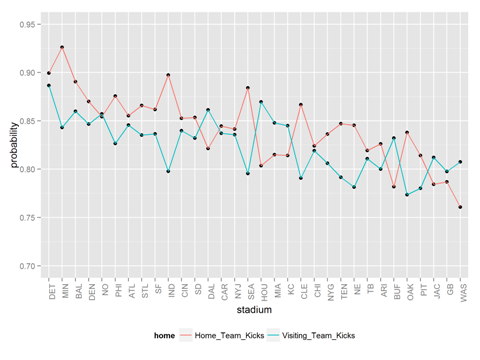
Click to enlarge
ADDENDUM 2:
Owing to the generosity of the great reader / Scottish economist Adam Smith (see comments), we now have the stadiums broken down by door (indoor or outdoor). The gray stadiums are either convertible (Houston) or have a small hole in the roof (Dallas).
Correlation is not causation, but it sure does seem plausible that having an indoor stadium helps the kicker.
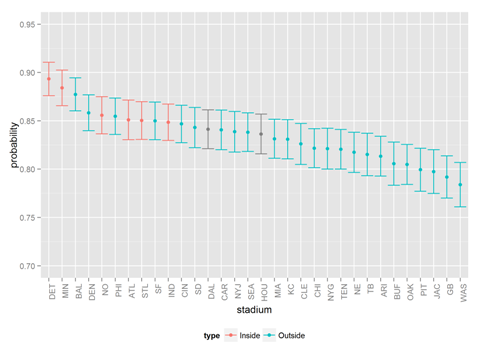
Click to enlarge
APPENDIX
To decode the team names, use this list:
ARI: Arizona Cardinals
ATL: Atlanta Falcons
BAL: Baltimore Ravens
BUF: Buffalo Bills
CAR: Carolina Panthers
CHI: Chicago Bears
CIN: Cincinnati Bengals
CLE: Cleveland Browns
DAL: Dallas Cowboys
DEN: Denver Broncos
DET: Detroit Lions
GB: Green Bay Packers
HOU: Houston Texans
IND: Indianapolis Colts
JAX: Jacksonville Jaguars
KC: Kansas City Chiefs
MIA: Miami Dolphins
MIN: Minnesota Vikings
NE: New England Patriots
NO: New Orleans Saints
NYG: New York Giants
NYJ: New York Jets
OAK: Oakland Raiders
PHI: Philadelphia Eagles
PIT: Pittsburgh Steelers
SD: San Diego Chargers
SEA: Seattle Seahawks
SF: San Francisco 49ers
STL: Saint Louis Rams
TB: Tampa Bay Buccaneers
TEN Tennessee Titans
WAS: Washington Redskins
Figure 1 Data:
Stadium Miss Hit
ARI 67 292
ATL 45 257
BAL 45 322
BUF 61 253
CAR 51 269
CHI 64 295
CIN 53 293
CLE 56 266
DAL 53 281
DEN 50 303
DET 34 285
GB 72 274
HOU 53 271
IND 55 308
JAC 64 252
KC 58 285
MIA 58 286
MIN 35 267
NE 63 282
NO 48 285
NYG 59 271
NYJ 50 260
OAK 72 297
PHI 51 300
PIT 65 259
SD 48 258
SEA 55 285
SF 51 289
STL 50 284
TB 58 256
TEN 63 288
WAS 70 254
Figure 2 Data:
ARI Home_Team_Kicks 32 152
Visiting_Team_Kicks 35 140
ATL Home_Team_Kicks 24 142
Visiting_Team_Kicks 21 115
BAL Home_Team_Kicks 23 187
Visiting_Team_Kicks 22 135
BUF Home_Team_Kicks 36 129
Visiting_Team_Kicks 25 124
CAR Home_Team_Kicks 23 125
Visiting_Team_Kicks 28 144
CHI Home_Team_Kicks 32 150
Visiting_Team_Kicks 32 145
CIN Home_Team_Kicks 28 162
Visiting_Team_Kicks 25 131
CLE Home_Team_Kicks 20 130
Visiting_Team_Kicks 36 136
DAL Home_Team_Kicks 30 138
Visiting_Team_Kicks 23 143
DEN Home_Team_Kicks 23 154
Visiting_Team_Kicks 27 149
DET Home_Team_Kicks 17 152
Visiting_Team_Kicks 17 133
GB Home_Team_Kicks 39 144
Visiting_Team_Kicks 33 130
HOU Home_Team_Kicks 32 131
Visiting_Team_Kicks 21 140
IND Home_Team_Kicks 19 166
Visiting_Team_Kicks 36 142
JAC Home_Team_Kicks 36 131
Visiting_Team_Kicks 28 121
KC Home_Team_Kicks 29 127
Visiting_Team_Kicks 29 158
MIA Home_Team_Kicks 32 141
Visiting_Team_Kicks 26 145
MIN Home_Team_Kicks 11 138
Visiting_Team_Kicks 24 129
NE Home_Team_Kicks 30 164
Visiting_Team_Kicks 33 118
NO Home_Team_Kicks 22 129
Visiting_Team_Kicks 26 156
NYG Home_Team_Kicks 27 138
Visiting_Team_Kicks 32 133
NYJ Home_Team_Kicks 26 138
Visiting_Team_Kicks 24 122
OAK Home_Team_Kicks 29 150
Visiting_Team_Kicks 43 147
PHI Home_Team_Kicks 25 176
Visiting_Team_Kicks 26 124
PIT Home_Team_Kicks 34 149
Visiting_Team_Kicks 31 110
SD Home_Team_Kicks 23 134
Visiting_Team_Kicks 25 124
SEA Home_Team_Kicks 19 145
Visiting_Team_Kicks 36 140
SF Home_Team_Kicks 25 156
Visiting_Team_Kicks 26 133
STL Home_Team_Kicks 22 142
Visiting_Team_Kicks 28 142
TB Home_Team_Kicks 30 136
Visiting_Team_Kicks 28 120
TEN Home_Team_Kicks 28 155
Visiting_Team_Kicks 35 133
WAS Home_Team_Kicks 39 124
Visiting_Team_Kicks 31 130
Figure 3 Data: See Figure 1 data plus the comments
Graphs were made in R using Hadley Wickham’s ggplot2 package. Pointer to the data can be found at our previous post.
Filed in
Jobs
Subscribe to Decision Science News by Email (one email per week, easy unsubscribe)
Filed in
Ideas ,
R
Subscribe to Decision Science News by Email (one email per week, easy unsubscribe)
EVERY NFL FIELD GOAL SINCE 2002
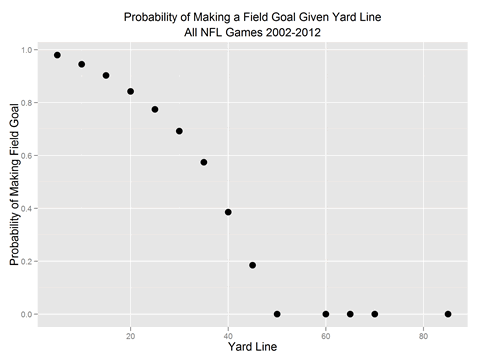
Click to enlarge
ADDENDUM: This Decision Science News post was covered by Bloomberg TV!
We looked at NFL punts before on Decision Science News. That’s old news. Field goals are the new hotness and Super Bowl Sunday is coming up, so let’s look at a kicker’s chances.
We’ve taken the same database and looked at the probability of getting the ball through the uprights depending on the yard line from which the kick starts. The result is above.
O data, you are so beautiful sometimes.
Those in need of a handy formula for the sidelines might want to use the following approximation. Let D be the yard line you are on:
- If D>50, you will miss
- Otherwise, your probability of making a field goal is about 1-.0004*D^2
We tried to fit a logit model, but it wasn’t pretty.
Speaking of beauty, one big data lesson is that data are more beautiful when binned. Here’s the more raw version:
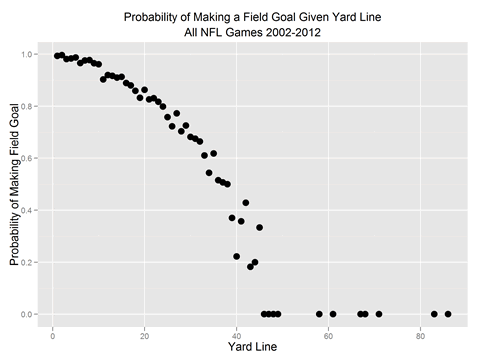
Click to enlarge
It seems coaches are aware of the maximum distance at which they have a prayer of making a field goal, and don’t even try otherwise:
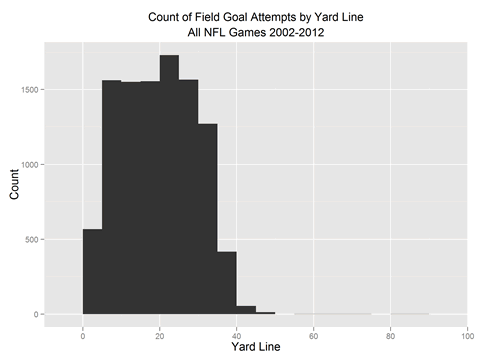
Click to enlarge
Graphs were made in R using Hadley Wickham’s ggplot2 package. Pointer to the data can be found at our previous post.
ADDENDUM:
Reader Anders made a chart that looks at the count of good and bad field goals by distance (yard line + 17):

Click to enlarge
ADDENDUM
We changed the model we had originally posted for one with just 1 free parameter. Here’s the fit:
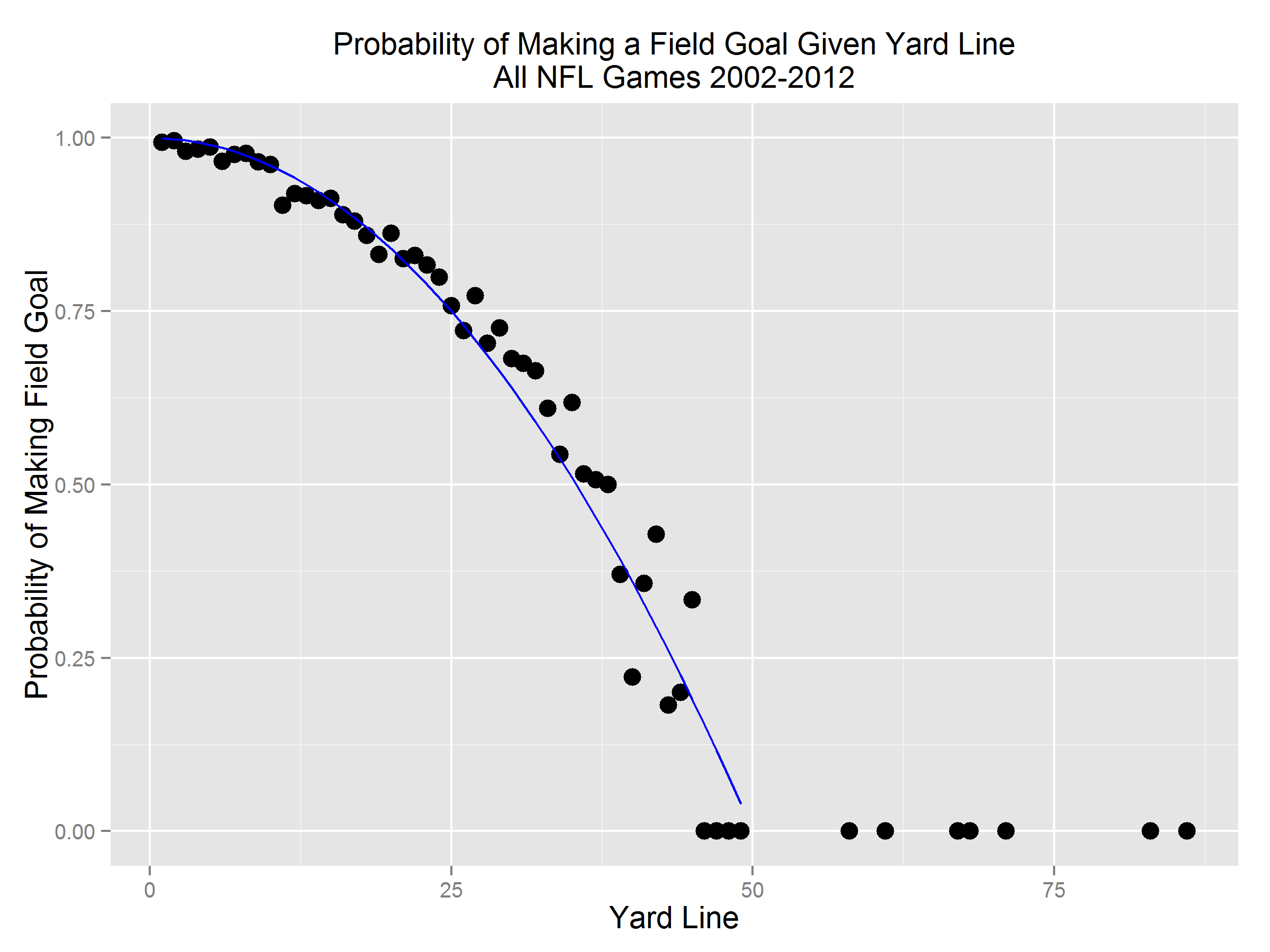
Click to enlarge
ADDENDUM
Some folks have asked if some of the longer attempts are noise. It appears so. Here are the descriptions of the attempts from behind the 50 yard line. On a regular attempt, the distance of the field goal is the yard line plus 17, so the case where the yard line is 86 and the field goal distance is suppose to be 68 yards, for example, seems to be a typo.
ydline |
description |
58 |
(:01) S.Janikowski 76 yard field goal is No Good Center-J.Condo Holder-S.Lechler. A.Cromartie at SD 2 to SD 28 for 26 yards (T.Stewart). |
86 |
N.Rackers 68 yard field goal is No Good Holder-D.Johnson. R.Droughns at NYG 2 to NYG 31 for 29 yards (T.Castille). |
83 |
M.Crosby 69 yard field goal is No Good Short Holder-M.Flynn. |
86 |
R.Bironas 58 yard field goal is No Good Holder-C.Hentrich. P.Buchanon at HST -6 to HST 29 for 35 yards (R.Reynolds). Penalty on HST-C.Anderson Offensive Holding declined. |
67 |
(:05) W.Richey 51 yard field goal is No Good Center-J.Maese Holder-D.Zastudil. D.Townsend at PIT -5 to BLT 45 for 60 yards. Lateral to J.Farrior to BLT 35 for 10 yards (T.Heap). FUMBLES (T.Heap) RECOVERED by BLT-T.Jones at BLT 42. T.Jones to BLT 42 for no gain (J.Gildon). |
61 |
(:01) J.Elam 57 yard field goal is No Good Center-M.Lepsis Holder-T.Rouen. C.McAlister at BLT -7 for 107 yards TOUCHDOWN. |
71 |
(1:46) T.Peterson 48 yard field goal is No Good Center-D.O’Leary Holder-T.Maddox. I.Bashir at IND -9 to IND 37 for 46 yards (R.Bailey). |
68 |
(:01) J.Hanson 50 yard field goal is No Good Center-B.Banta Holder-J.Jett. D.Sharper at GB -9 to GB 35 for 44 yards (R.Brown). |
Filed in
Conferences
Subscribe to Decision Science News by Email (one email per week, easy unsubscribe)
24TH SUBJECTIVE PROBABILITY, UTILITY, AND DECISION-MAKING CONFERENCE

The European Association for Decision Making invites you to attend its next biannual
24th Subjective Probability, Utility, and Decision Making Conference (SPUDM
24), which will be held at IESE Business School – University of Navarra in Barcelona,
Spain, on August 18-22, 2013.
Submissions of paper abstracts, poster abstracts, and proposals for workshops are
invited on any topic in basic and applied judgment and decision making research.
Deadline for all submissions is March 8, 2013.
The organizing committee is pleased to announce that the conference will feature the
following invited speakers:
• Timothy D. Wilson, University of Virginia, USA
• Colin F. Camerer, California Institute of Technology, USA
• Robin Hogarth, Universitat Pompeu Fabra, Spain
• Ralph Hertwig, Max Planck Institute for Human Development, Germany
The call for papers is available at:
http://www.iese.edu/en/events/OtrosEventos/SPUDM24/Home/Home.asp
Attending this meeting will also be an opportunity to discover Barcelona, one of the
most unique and architecturally distinctive cities of the world. Barcelona is the capital
of Spain’s Catalan region, which has produced a number of the world’s most prominent
artists including Pablo Picasso and Salvador Dalí. The architect Antoni Gaudí also left
his indelible mark on the city through a number of remarkable buildings such as La
Sagrada Familia, La Pedrera, and La Casa Batlló.
We look forward to seeing and welcoming you in Barcelona!
The local organizing committee:
Elena Reustkaja (IESE, Spain)
Mario Capizzani (IESE, Spain)
Franz Heukamp (IESE, Spain)
Robin Hogarth (UPF, Spain)
NEW DECISION JOURNAL FOCUSES ON THEORETICAL ISSUES
Good news for Judgment and Decision Making researchers: a new APA journal entitled Decision is getting ready to launch. Be on the lookout for its web site in the next weeks. Here are some details.
Decision
A journal for research on judgment and decision making
Decision is a multidisciplinary research journal focused on a theoretical understanding of neural, cognitive, social, and economic aspects of judgment and decision-making behavior. Decision will publish articles on all areas related to judgment and decision- making research including probabilistic inference, prediction, evaluation, choice, decisions under risk or uncertainty, and economic games. The journal will publish articles that present new theory or new empirical research addressing theoretical issues or both. To achieve this goal, Decision will publish three types of articles: long articles that make major theoretical contributions, shorter articles that make major empirical contributions attacking important theoretical issues, and brief review articles that target rapidly rising theoretical trends or new theoretical topics in decision making.
Editorial Board
Colin Camerer
Nick Chater
Peter Dayan
Gerd Gigerenzer
Reid Hastie
Mark Machina
Amnon Rapoport
Roger Ratcliff
Valerie Reyna
Jim Sherman
Richard Shiffrin
Josh Tenenbaum
Joyce Wang
Thomas Wallsten
Angela Yu
Filed in
Ideas ,
R
Subscribe to Decision Science News by Email (one email per week, easy unsubscribe)
ANALYSIS OF THE PUNTING DECISION BASED ON 11 YEARS OF PLAY-BY-PLAY DATA
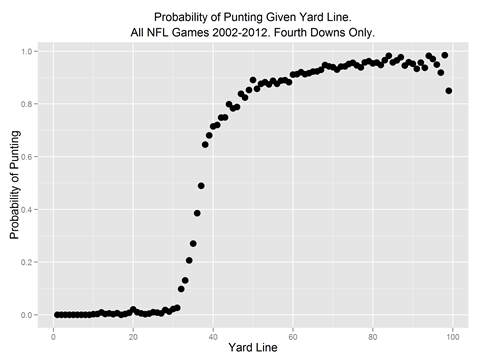
CLICK TO ENLARGE
The site reddit told us about data on every single NFL (U.S. National Football League) play since 2002. We read it in and did an analysis of punting. The results are beautiful.
North Americans metaphorically say they’re “going to punt” and people not familiar with American and Canadian Football can be unclear about what they mean. In the UK, “punt” means many things (such as pushing a boat with a stick or visiting a prostitute), but not the North American metaphorical sense.
Loosely put, to punt means to play it safe by not trying something risky that could leave you worse off. In football, it’s kicking the ball into a better field position instead of staying where you are and fighting because if you stay and fight you might lose and your opponent will have you in a bad field position. You punt when your back is to the wall, on your last “down” or opportunity to do something before the opponent automatically gets the ball. Higher risk alternatives to punting are passing or running with the ball. When close to the opponent’s goal, an alternative is trying to kick a field goal. [I realize that’s a bad description but maybe we can crowdsource a better one].
We made some graphs of the punting decision and found some beauty in these strategic behavior data.
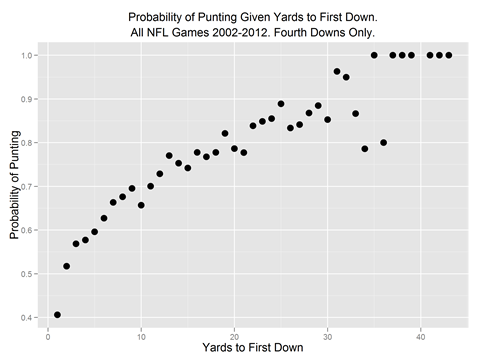
CLICK TO ENLARGE
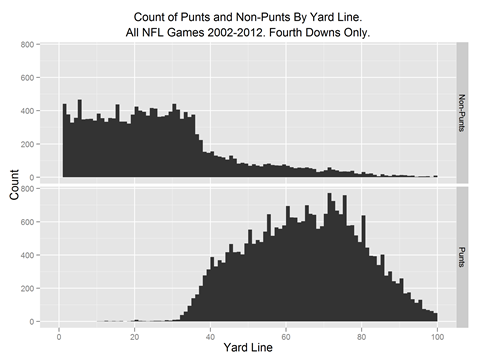
CLICK TO ENLARGE
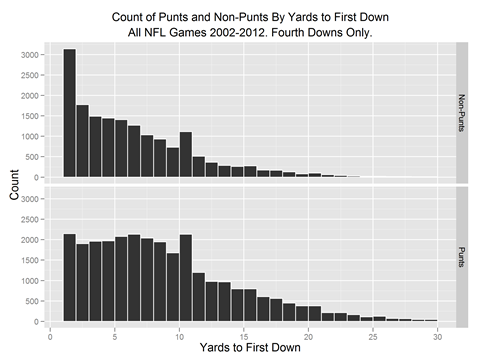
CLICK TO ENLARGE
Thanks to the reddit post and Advanced NFL Stats, as well as to the open source R language and Hadley Wickham‘s ggplot2.